Open data in Scotland: a blueprint for unlocking innovation, collaboration and impact
This independent report was commissioned by the Scottish Government
Digital Directorate on the current international and domestic position on open data. This has been used to inform high-level recommendations on next steps for open data in Scotland.
2. Open data: literature review
2.1 Defining open data
There is relative unanimity about what ‘open data’ and ‘open government data’ mean. Open Knowledge Foundation’s widely used Open Definition[2] states that open data is:
“[Data that] anyone can freely access, use, modify, and share for any purpose (subject, at most, to requirements that preserve provenance and openness)”
Open government data is any data produced, collated or commissioned by public bodies, which conforms to this definition or an equivalent open definition.[3]
This simple definition can be expanded upon in two main directions. One approach is output-based, using the legal and technical characteristics of data to define its openness. The full Open Definition takes this approach, requiring open data to be:
- Published under an open license[4]
- Accessible for free or at cost
- In a machine-readable format
- In an open format
The other approach is process-based, and judges the openness of data on the principles, policies and processes that shape data governance and publication. Examples of this contextual approach include the original 2007 Open Government Data Principles[5], which included requirements that data be timely, complete and granular, and the wider concept of Open ICT for Development, which stressed universal access and participation and collaborative production methods.[6]
The output-based definition of open data is now dominant but questions over process and context remain relevant, even if they are now approached via a data governance and strategic lens (see sections 2.2 and 2.3).
2.2 Trends in open data
The term open data was coined in 1995, in reference to the free exchange of scientific data. The Open Government Data Principles were written in 1997, with the US adopting a transparency and open government directive in 2009, and the first International Open Data Conference taking place in 2010. Open data has since become increasingly important to policy initiatives across the world, as a transparency mechanism, a practical tool to tackle policy issues, and as a means to measure and monitor progress on other goals.[7]
Underneath this narrative however the priorities and methodologies of the open data movement have changed significantly. Verhulst et al. describe several chronological ‘waves’ of open government data that act as a useful framework[8]:
- First Wave: data is released piecemeal in response to requests based on freedom of information laws.
- Second Wave: data is released using an "open by default" principle, based on the idea that open data will catalyse innovation, evidence-based decision-making, civic engagement, and a culture of collaboration. Limited attention is paid to demand during this phase. Scotland currently has “open by default” as a principle of its own open data strategy.
- Third Wave (current phase): a purpose-driven approach supplants the sometimes scattershot publication of the Second Wave. This prioritises impactful reuse and broader collaboration to produce complex datasets needed to address complex challenges.
- Fourth Wave (predicted): generative AI makes it easier to use and analyse data, improve data quality, and allow the creation of synthetic datasets where privacy or availability is a concern.[9]
One way to interpret the ‘waves’ of open government data is as an evolution of the motivation for publication from:
- A compliance approach, driven by reactive responses to external stakeholders.
- A principles approach in which openness is integrated into the data governance principles used by public bodies.[10]
- An intentional approach, in which open data publication is planned and targeted to align with other policy priorities and to fit the resource and capability constraints faced by publishers.
All three of these attitudes can be found among open data stakeholders in Scotland.
The transition from the second to third wave in the public sector has been and continues to be driven by:
- An increased public awareness and literacy around data availability and use;
- Improved understanding about why and how data practitioners can be embedded in the broader processes and governance structures of local communities directly affected by open data projects; and,
- A targeted, demand-driven approach as a more practical and sustainable option for organisations with limited financial and technical resources.
The following case studies are examples of the third wave approach to open data.
Case study 1: Scotland’s financial transparency Open Government Partnership (OGP) commitments
Purpose
- Improve reliability and accessibility of fiscal data, so that a wide range of users can understand and reuse it.
Partnerships
- Extensive collaboration within and between departments, civil society and domain experts.
Approach
- Identification of high value and high impact datasets. Making long-term changes to improve access via stakeholder engagement and technical improvements.
Impact
- Procurement transparency with data published in line with the Open Contracting Data Standard (OCDS).
- Standardisation and visualisations of extensive disclosures on infrastructure investment.
- Ongoing work on improved transparency around the Scottish Budget.
Case study 2: International Aid Transparency Initiative (IATI)
Purpose
- Meet transparency commitments set out in the Accra Agenda to help a diverse range of stakeholders improve the effectiveness and efficiency of aid and development finance.
Partnerships
- Providers of development assistance, partner countries, multilateral institutions, private sector and civil society organisations and others.
Approach
- Shift from general disclosure to the targeting of data disclosures and data use initiatives for specific purposes.
Impact
- Interactive map of the African Development Bank’s investments in Africa helps with allocation of resources.
- Aligning international aid flows with national priorities improves government decision-making in Liberia.
- Sectoral initiatives to understand where aid is going and how effective it is (eg. Pacific Aid Map, DFI investments in gender, global air quality funding).
While the Scottish Government is shifting to a more intentional approach to produce complex and connected datasets, civil society is largely driven by a principles approach in which data should be open by default, and many smaller public sector organisations see open data as a compliance issue. This diversity of approaches is almost inevitable but it can lead to misunderstandings and uncertainty if unaddressed.
2.3 The value and impact of open data
Value and impact are ultimately why public sector organisations publish open data. Understanding and documenting the expected value and impact of open data can help build support for open data, feed into choices over what and when to publish, and measure outcomes. This section describes some of the most important ways to think about the impact and value of open data and outlines the most common techniques for measuring that impact and value.
2.3.1 Impact
The expected impact of open data can take many different and overlapping forms.
- Transparency, accountability and empowerment. Open data can support transparency and accountability when it is used as a public record of actions taken by public actors and institutions. Open data improves accountability because it allows the public to engage with and understand such disclosures (and for public bodies to respond).[11] Open data can help citizens to be more engaged and empowered by allowing direct access to information about local communities and areas of interest, and by creating the infrastructure for accessible information services aimed at non-technical audiences. Examples include:
- Open budget, spending and procurement data have made complex public sector finances more accessible and comprehensible.
- Registers of political interests and information on elections and parliamentary activity have allowed citizens to engage more closely with democratic processes.
- Open data about local services, facilities and socio-economic indicators has improved the ability of citizens to understand and engage with local issues.
- Innovation and economic growth. Open data can contribute to innovation and economic growth, primarily through datasets that directly form part of value-added activities where data is the primary product or service. These include enhancing, interpreting or combining data. Data sets may also indirectly support value-added activities where data is not the primary product or service. For example, these allow innovations in processes, improving market function, providing efficiency gains or reducing risk. Examples include:
- Open transport datasets led to passenger information and mapping services.[12]
- Open company and financial information led to due diligence and compliance services.[13]
- Open satellite mapping increased the rate of gold discoveries and the share of the market held by new entrants.[14]
- Open data on public and publicly-funded services has made it easier for end-users and intermediaries to find suitable services.[15]
- Management and monitoring. Open data can support the management and monitoring of public policy goals by providing indicators of progress derived from existing datasets, and by identifying and filling gaps in current data collection and generation. Examples include:
- Scotland’s National Performance Framework relies on multiple open data sets to provide visibility on progress towards agreed outcomes.[16]
- Shortcomings in available gender data to monitor progress towards the Sustainable Development Goals (SDGs) has led to a movement to improve funding for gender-based data systems.[17]
- Collaboration and coordination. Open data can improve collaboration and coordination around public policy goals by enabling data to be shared across government with a minimum of friction, and allowing data to be shared and collated across the public, private and third sectors. Examples include:
- Publication on financial commitments, transactions and results of international development and humanitarian activities has allowed providers and recipients of assistance to better plan, evaluate and coordinate their work.[18]
- Sharing and publication of data on climate and natural disaster risks allows governments, scientists, local communities and private sector stakeholders to better plan, maintain and protect infrastructure and physical safety.[19]
2.3.2 Value
Similarly, there are many different models for thinking about the value of open data. Maximising the value of open data means realising multiple types of value, rather than pigeon-holing data into one particular area. Examples include:
- Social and economic value. Some data is designed for explicitly social ends (for example, data on democracy or about local communities), while other data is published primarily for its potential impact on the economy. For example, mapping and transport data. Over time new use cases will tend to blur these distinctions. There is an ecosystem of due diligence and analytical companies built around open company data, for example, but this data is also widely used by civil society organisations working on integrity or environmental issues. Similarly, 360Giving, which allows open data on grant funding, realises value across this spectrum: providing transparency on the equitability of the sector; helping grant-makers to collaborate to be more effective and intentional; and, making the process of finding grant funding more efficient.[20]
- Planned and emergent value. For some open data the value of publication might be understood up front, or clarified during the research and design phase, as part of the kinds of intentional and planned publication associated with the third wave of open data. This is particularly relevant for complex and collaborative datasets, where the investment needs to be justified and the outputs need to meet specific and well understood user needs.[21] But the value of open data can also emerge over time, as new use cases are developed. In this case it may be preferable to reduce the time spent on planning and design and focus instead on making data accessible, discoverable and easy to use.[22]
- Primary and secondary value. The most obvious type of value stems from direct or indirect use of open data, as new activities and services are enabled or improved. But the process of producing open data, and the culture it encourages, can also create positive spillover effects, like improvements to data governance and IT processes, or the creation of new working relationships within and outside the public sector.[23] These types of a secondary value can be a conscious by-product of ‘open data as strategy’, in which the approach required to produce useful open data is valued on a par with the technical outputs.[24]
- Wide value and narrow value. Another difference exists in the scale and breadth of value that an open dataset delivers (or is expected to deliver). The concept of ‘high value datasets’ used in the EU Open Data Directive, for example, is based on open data having significant impacts for large numbers of beneficiaries.[25] But significant value can also emerge from ‘highly valued datasets’ that are of vital interest to a smaller audience.[26]
- Output and outcome value. Finally, value can be seen in terms of outputs (for example, the number of datasets produced and the technical quality of those datasets) and also in terms of outcomes (what the underlying information allows direct or indirect users to achieve). Large quantities of well-ordered, high-quality data is the ideal situation but, nonetheless, significant value can be derived from poor data of dubious quality when users embrace the ‘messiness’ of such information. For example, the Organised Crime and Corruption Reporting Project’s Aleph software is designed to help journalists and investigators navigate the complexity of official and unofficial records of corporate activity.[27]
2.3.3 Measurement
Measuring the value and impact of open data is important to build and retain support for publication and to make sure that plans are informed by evidence about what works. That said, measurement is recognised as difficult and detailed evidence on impact remains scarce.[28] The main ways in which value and impact can be measured are:
- Qualitative case studies of particular data initiatives or areas of impact. These can be particularly useful in making the value of open data clear to non-technical audiences and in identifying priority areas for improvement. Case studies are vulnerable to cherry-picking, however, and so are likely to work best when produced by sector experts and end users who can provide honest feedback.
- Quantitative case studies that use economic modelling or other methods to estimate the benefits of open data.[29] These can be particularly useful to overcome scepticism over potentially complex and costly publication and to make cost-benefit analysis before and after publication.[30] It can be difficult to isolate the impact of open data in economic modelling, however, and there is no one-size-fits-all methodology so this can be a complex approach.
- Quantitative indicators that demonstrate how open data is being used can be useful to understand impact over time and to integrate open data initiatives into existing Monitoring, Evaluation and Learning frameworks. While potentially useful, such indicators can be difficult to design, and there may be a temptation to choose unambitious targets if they are used to measure success or failure.[31]
- Surveys and catalogues of data use and impact among stakeholders can be useful to understand the uptake and usage of open data inside and outside the public sector. The GovLab has collated a collection of global impact case studies and Ireland uses a regular survey to gauge the use of open data.[32]
2.4 Producing, using and achieving impact with open data
The optimal practical approach to producing open data has also changed as the field has matured because publication is now recognised as a socio-technical problem—that is a problem with ‘organisational, human, material, and technological’ dimensions.[33] This means that, while sound technical implementation remains fundamental, the environment in which open data is published is seen as a key limiting and enabling factor.
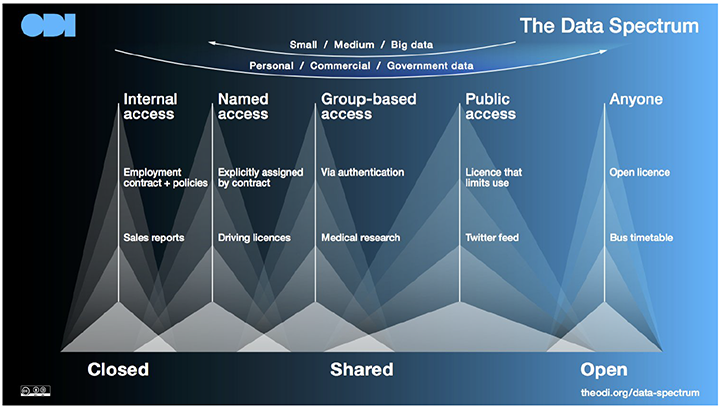
Source: the Open Data Institute, under a CC-BY license
Rather than a narrow focus on purely open data, we can derive benefits from sharing data in different ways (see figure 1, above), which range from granting limited access, (such as, by restricting the audience or redacting parts of the dataset) to full access for reuse under an open licence. Sharing and opening data can be mutually reinforcing, as many of the organisational, technical, and policy barriers are the same.
This section discusses the idea of data value chains (which incorporate the full complexity of open data publication, broadening the discussion to beyond the lifecycle of just the data itself), some of the most important data governance principles for open data, and the implications of this for technical guidance about open data.
2.4.1 Data value chains
An open data value chain is the set of activities that lead to the identification, publication and use of open data, designed to maximise the impact of open data at each stage in the open data lifecycle. An open data value chain can be a design tool, allowing the right activities to be identified to maximise impact, and a diagnostic tool, allowing points of friction and areas for improvement to be identified.[34]
Figure 2 below shows an example open data value chain with some non-exhaustive example activities at each of the four main stages.
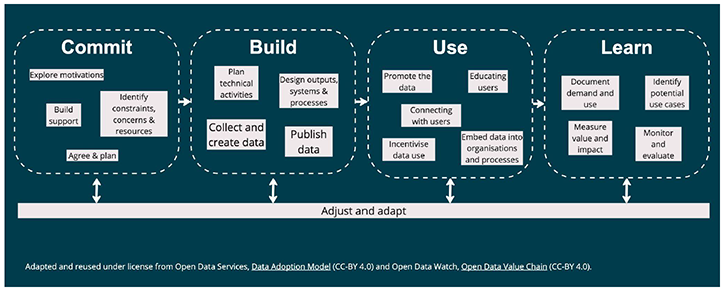
Source: Adapted and reused under license from Open Data Services, Data Adoption Model (CC-BY 4.0) and Open Data Watch, Open Data Value Chain (CC-BY 4.0)
An open data value chain is a lens through which to view the full lifecycle of open data, focusing on the impact - potential, or realised - of each stage.
- Commit: This stage involves getting to an agreement to publish. This may involve implementing an existing policy or directive, or building support for a new initiative. This stage also incorporates important design elements, such as defining the purpose of publication, setting data governance principles and policies, identifying key stakeholders and building partnerships, and understanding the likely material, organisational or human constraints.
- Build: This stage involves the activities that lead to data publication itself, including data collection and mapping, technical design and negotiation, designing and implementing data governance practices, creating data pipelines, and publishing and cataloguing the data.
- Use: This stage involves turning published data into a resource that is actively used and understood. Relevant activities may include data analysis itself, promoting data to potential users, forging partnerships, and capacity-building for potential users.
- Learn: This stage involves understanding how data is being used, the impact it has and any areas where potential is not being fulfilled, or where implementation issues are holding back impact.
There are three overarching concepts that apply to all open data value chains:
- Iteration: A data value chain is not linear and there is no definitive endpoint. Instead, adaptation and adjustment should take place in parallel and learning from each stage should feed back into other stages.
- Collaboration: Open data value chains are collaborative by nature. They bring together technical and non-technical stakeholders and stakeholders from across the public sector with civil society and the private sector. Stakeholders’ interests and levels of involvement will vary across the open data value chain. A well-functioning open data value chain will result in coordinating the needs and challenges of these different groups.
- Context: The design of an open data value chain is highly context-dependent. This context covers multiple levels, from the high-level of the legal, regulatory and policy environment, to the constraints and priorities of implementing organisations, and down to the skills and interests of teams and individuals. There is no off-the-shelf solution for open data publication and an appropriate open data value chain will be tailored to specific needs.
Finally, open data value chains are useful for highlighting the complexity of data publication and therefore the many ways that expected impact can fail to materialise if implementation is not done well.[35] Critical reflection on activities across the full open data value chain can be a useful diagnostic tool in identifying and fixing issues with key activities.
2.5 What do we mean by ‘guidance’ for open data
This research was originally designed to provide advice on ‘technical guidance’ for open data. As the previous sections have shown, however, open data publication is much more than just a technical problem and a wider concept of guidance is required.
The core purpose of open data guidance should be to help publishers design and navigate the technical and non-technical activities in a data value chain, reducing friction and identifying key touch points that can maximise positive impact.
This section discusses three layers of guidance that can achieve these goals: creating an enabling environment for open data; setting the high level direction for open data publication; and providing tools, resources and support. In Section 4, we discuss which of these are practical and realistic for the Scottish Government to provide.
2.5.1 An enabling environment
The impact of detailed guidance on open data publication will depend on the quality of the enabling environment in which they sit. Three elements of that enabling environment, which policy initiatives can help improve, are particularly relevant:
- Direction and leadership. Strong direction and ongoing leadership on the importance of openness and open data can help motivate publication for public sector organisations, set expectations on what will be published, and reduce the perceived risks of transparency and data publication. Detailed open data policies (or, in certain circumstances, legal and regulatory requirements) can further reduce uncertainty, allowing organisations to then concentrate on implementation. While the Scottish Government has numerous commitments to open data the perception is that the ambition is relatively low and implementation lags behind, in part due to misaligned or inappropriate expectations, and a lack of high-level leadership in the area.
- Culture and trust. A culture aligned with the principles of openness and collaborative working is important, particularly when considering how to move beyond a risk-averse, compliance mindset. An open culture built on trust can help with several key challenges: creating genuine enthusiasm around the benefits of transparency, building cross-sector partnerships designing initiatives with the input and feedback of stakeholders, and seeing open data publication as a collaborative and collective commitment in which the public sector, the private sector and civil society need to work together. While the Scottish Government is committed to participatory and open principles there is a perception that these are not universally held across the public sector and the relationship between data publishers and civil society can sometimes feel combative rather than collaborative.
- Capability and resources. Closing the gap between ambition and implementation relies on public sector organisations having the capability to publish data, and end users having the capability to analyse, understand and deploy data. The distributed responsibility for publication in Scotland (see Section 3.2) makes this a difficult problem as organisations often have very limited technical resources and are starting from scratch on open data. These same problems also apply to end users, who may be interested in the information that has been published, but lack the skills or time to process technical outputs. These deficits may be tackled via a general and long-term approach to improving digital skills and data literacy, the provision of some central technical resource, or by encouraging ‘infomediaries’ to contextualise and explain open data to non-technical audiences.[36]
2.5.2 High level direction
Setting the high level direction via a common data governance framework for publishing open data can help remove uncertainty about what data needs to be published, how that data should be published and managed, and the quality and technical standards that are expected. This reduces the planning and decision burden on data publishers, makes the data more predictable and usable for end users, and creates shared expectations on data availability, quality and other factors.
Some particularly relevant aspects of data governance for open data in Scotland include: policies, strategies, principles, quality standards, technical standards, discoverability and availability.
Open data policies are a common tool for establishing the ground rules for publication and can create a solid foundation for impactful data publication and use. The most important elements of such a policy include setting an overarching purpose, specifying a justification for publication (including via a legal framework), establishing operational approaches, specifying quality and technical standards, assigning responsibility for key tasks, providing a framework for producer-user relationships, and discovering and stimulating demand and supply.[37] A strategy can be useful for operationalising a policy, avoiding the risk that a policy remains a paper exercise only. South Korea, for example, established an open data law in 2013, backed by regular high-level plans that encourage a purpose-directed approach to publication and a more granular plan that directs data releases.[38] The Scottish Government has a number of policy initiatives related to open data but has been less successful in establishing shared expectations on the practicalities among publishers, leading to large divergences in practice and a publication landscape that is generally fragmented and confusing for end users (see Section 2 and 3).
A set of principles can help guide both high-level decision- and policy-making and implementation and planning at a more granular level. These principles can cover planning and strategic decisions, the quality of data outputs, management of data assets, and ways of working (such as clear communication and taking a consultative and participative approach).
Upholding data quality standards can reduce the burden on end users, improve uptake of data and increase confidence in data. Data quality can be approached from the perspective of:
- Broad principles and frameworks, to set out the criteria for managing data well, narrowing down options in areas such as technologies used, licensing, metadata, archival. Principles tend to work in multiple contexts, so a context-specific ‘profile’ of a set of principles can be helpful for guiding implementation and measuring progress. Examples include: the UK Government Data Quality Framework, the FAIR Principles[39]; the W3C Data on the Web Best Practices.
- Macro measures, for assessing data quality at a national or organisational level, across multiple axes, including accessibility, coverage, completeness. Examples include: the European Data Maturity Report; the Global Data Barometer; surveys of data published in a particular region such as that of available open data in Scotland produced by Code the City.
- Micro measures, which can be a powerful tool for assessing the quality of individual datasets, ranging from generic measures of technical quality to very targeted and domain-specific measures informed by specific user needs. Examples include: the 5* Open Data framework[40]; bespoke tools for particular data standards[41].
Using technical standards can reduce the burden on data consumers, and make data publication easier (particularly if relevant support and resources are available). Technical standards can cover:
- Using common formats and access methods, which make open data more accessible and useful to end users, who can integrate data into existing workflows and use common tools and software libraries. Examples include: CSV, JSON and JSON Schema, the UK Government list of approved technical formats for the exchange of information; the FAIR Principles suggest the use of common and open formats and protocols for publishing and exchanging information; the Open Geospatial Consortium library of standards for interoperable geographic information; Open API; the Realtime Paged Data Exchange (RPDE) format.
- Metadata (data about data), which is essential for providing context for published data and improving both automated and manual discoverability. Metadata can be used to provide information on the content, format, themes, status, ownership and provenance of datasets, and can greatly improve the trustworthiness and usability of published data. Examples include: DCAT (Data Catalog Vocabulary) (Data Catalog Vocabulary); EU Metadata Quality Assessment.
- Data standards, to provide an agreed format and requirements for publishing data on specific topics. Data standards enable interoperability and exchange between systems, promote comparability between datasets, and encourage publication of data by making it easier to prepare and share data. Data standards are particularly useful for describing data in complex fields or where collaboration and coordination are desired outcomes. [42] Examples include: 360Giving (for grants), Open Referral (for human services), International Aid Transparency Initiative (for humanitarian and development assistance) Open Contracting (for procurement) and Infrastructure Transparency Initiative (for infrastructure).
- Using common components and formats to aid interoperability and reuse, particularly for high value fields like organisational identifiers or very common fields like dates (e.g. ISO 8601) and monetary values. Using shared concepts and definitions reduces the burden on publishers (who don’t have to invent a methodology or definition from scratch) and on users (who don’t have to disentangle the meaning of a field on a per dataset basis). Examples include: org-id; Legal Entity Identifier (LEI); currency and country codes; geographic information and coordinate systems (for example, WGS84 coordinates); the Statistical Data and Metadata Exchange (SDMX) model.
There are also a variety of approaches to specifying technical standards, summarised below.
Discoverability and availability of data is critical if it is to be reliably found and used, both manually by humans and programmatically by machines. The most important approaches to making this happen include: the use of accurate metadata and other markup to describe datasets and improve search-based discoverability; clear policies around uptime and retention; and the use of centralised services to collate publications and provide context, signposting and quality control.[43] The Scottish Government uses the FAIR Principles, which are very useful but primarily concerned with technical quality and design. This leaves gaps around how to prioritise and plan data publication, how often data will be updated and how long it will be available for, and how publishers should interact with users. It is vital to ensure existing and future policies around data publication have buy-in from internal and external stakeholders, and to encourage uptake by integrating the practical aspects of these principles, along with expectations around quality and measuring progress, into organisational data strategies.
Discoverability and availability of data in Scotland is an area of weakness due to fragmentation, uneven publication policies among public sector bodies, a lack of signposting, and lack of reliable access and retention to data.[44] This is reflected in, for example, the fact that civil society created a grassroots data portal in the absence of an official alternative and that awareness of successful projects to publish complex data sets (for example, around procurement or fiscal data) remains low, even among open data enthusiasts. Discoverability is now being partially addressed via a CivTech challenge to provide accessible search and quality assessment of open data in Scotland.[45]
2.5.3 Tools, resources and support
Practical guidance in the form of tools, resources and support can help publishers identify and achieve key activities and help users engage with and generate impact from published outputs.
Information and documentation
Publishers and users both benefit from clear guidance on what open data is and the key processes and considerations involved in producing and using open data. These types of resources may include non-technical explainers, technical documentation and practical tools or templates to assist with common activities in the data value chain. This can take the form of:
- Briefings on the value of and requirements for impactful open data for non-technical audiences.[46]
- General open data toolkits that combine documentation and signposting to other resources.[47]
- Guidance on specific open data topics, such as licensing, anonymisation or identifiers.[48]
- Publisher-facing documentation of tools, operational processes and activities that are part of the data value chain.[49]
- User-facing documentation of datasets, access and analysis tools, and underlying methodologies.[50]
- A knowledge base that collects and documents common queries and provides guidance or context for using and interpreting open data.[51]
- Collating and signposting existing resources, which may otherwise be fragmented and difficult for users to discover, and by designing user journeys through such resources.
Tooling and infrastructure
Some central provision of technical resources, tooling and infrastructure to aid open data publication can provide significant savings, quality control and generally ease the road to publication. This can take the form of:
- Provision of a central data portal and/or publishing service to avoid fragmentation and divergence in practice.[52]
- Designing dedicated systems for analysing, visualising and explaining data on particular themes.[53]
- Resourcing software or software-as-a-service tools to make data publication or analysis easier.
- Providing data quality tools to identify and fix issues with the data or metadata.
- Using open source methodologies and licensing to promote the reuse of tools and techniques and give users visibility on changes.[54]
Direct, hands-on support
Expert support on publication can be very helpful, especially when responsibility for publication is distributed and organisations may lack the specialist skills or resources needed to meet their obligations. Publishing novel or complex datasets, or publishing to data standards, is another situation where direct support can be useful. This could involve:
- A helpdesk model where publishers and/or users can resolve queries and receive direct technical assistance.[55]
- Procurement of external resources to execute complex or novel data publication initiatives.
- Funding permanent public sector staff either directly in publishing organisations or to assist publication across a number of public sector organisations.
- Secondment of staff to organisations that lack experience or capacity to publish open data.
Community and capacity building
Open data publication works best as a collaborative endeavour. Guidance should reflect this by cultivating a supportive community that can reflect honestly on the challenges and find collective solutions. This can be achieved by:
- Developing standards and tools in open source repositories, where users can provide feedback and report issues.
- Creating and facilitating forums, or other online spaces, where publishers and users can share experiences, ask questions or provide feedback.[56]
- Hosting facilitated community calls or office hours where substantive issues can be discussed.[57]
- Offering training sessions and self-service training materials to improve understanding of specific issues or build skills.
Scotland already has many of the components of a useful support ecosystem in place. The challenge will be to iterate on these, while pulling them into a coherent and comprehensible package for data publishers and end users.
Contact
Email: martin.macfie@gov.scot
There is a problem
Thanks for your feedback