Offshore Wind Sustained Observation Programme (OW-SOP): scoping report
Physical processes are important as they influence the productivity of the phytoplankton which form the base of the entire North Sea ecosystem. This project recommends approaches to assess the potential impact of offshore wind farms on physical processes.
Appendix C. Supporting material (Chapter 3)
i. Induced drag and mixing of the underwater structures (fixed or floating)
The sub-grid parameterization of the foundation drag and wake turbulence has been described in Rennau et al. (2012), Carpenter et al. (2016) and Christiansen et al. (2023). It is based on an additional quadratic friction term in the momentum equations as well as additional production terms in the dynamic equation for TKE and its dissipation rate. In their method, the drag by a vertical cylinder perpendicular to an unstratified flow can be expressed as:
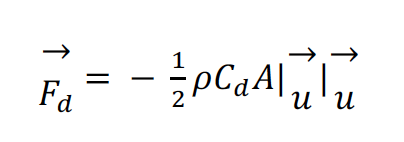
where ρ is the density of the fluid, Cd is the drag coefficient and A is the frontal area of the cylinder that is exposed to the free stream and u is the velocity of the free stream, with a negative sign indicating that the drag force is acting in the opposite direction of the free stream. Although the parameterization was developed for unstratified flow, Rennau et al. (2012) suggested that the basic principles of the approach also apply for stratified flow. To account for deceleration at grid cells containing offshore wind turbines, the drag retarding force has to be added to the hydrostatic momentum equation (with equations depending on the hydrodynamic model used).
In order to account for the production of sub-grid wake turbulence, the driving assumptions is that the turbulence produced by foundations is equal to the power lost to drag, Pd,
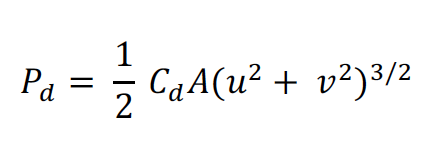
is then included as further production term in the TKE equation and as a source term into the dissipation rate as well, scaled by an empirical parameter c4. Rennau et al. (2012) derived the c4 parameter by calibrating an extended k-epsilon turbulence closure model by comparing LES simulations of stratified flow past 10 m diameter cylinders. The physical meaning of this parameter could be identified to be connected to the structure induced mixing efficiency. It was found that a value of c4 = 1.4 resulted in mixing slightly smaller than predicted (20% mixing efficiency) and c4 = 0.6 (50% mixing efficiency) resulted in an overestimation of mixing.
In general, the results of the parameterization of the foundation drag and wake turbulence are strongly dependent on the choice of scaling parameters. While Rennau et al. (2012), Carpenter et al. (2016) and Christiansen et al. (2023) assumed that Cd is constant in reality it is variable and dependent on the Reynolds number, surface roughness and structure geometry. The drag is also likely a function of depth and time and will vary with background shear and Froude number (Dorrell et al., 2022).
In terms of water column changes, Cazenave et al. (2016) compared hindcast scenarios without OWFs and with 242 fixed WTs installed in the eastern Irish Sea. Localised regions of decreased velocity extend up to 250 times the monopile diameter away from the monopile. Changes in tidal amplitudes were found to be up to 7%. The turbines enhance localized vertical mixing which decreases seasonal stratification, the horizontal extent of this disturbance is significantly larger than the sum of the footprint of the monopiles.
For seasonally stratified tidal flow, Carpenter et al. (2016) calculated that the loss of tidal energy to turbulence is between 4 - 20% of the energy loss due to bottom friction in the German Bight. The existing 160 fixed turbines in the German Bight in 2016 would not significantly decrease stratification there. However, extensive installations covering large parts of the German Bight could lead to a measurable large-scale reduction in stratification (Carpenter et al., 2016).
Christiansen et al. (2023) found that in the German Bight the additional turbulence production is the main driver of changes in both current velocities and stratification, with magnitudes of about 10%, similar in magnitude to regional annual and interannual variabilities. However, the magnitude of these changes should still be interpreted with caution: the choice of the drag parameters (Cd and c4) is decisive for the magnitude of the model results and lead to uncertainties in the local mixing of more than 100% with the drag coefficient having the largest influence. In general, more observations are needed to validate the modeling approaches and to support the simulation results. Nevertheless, the expected dimension of the structure-induced effects of offshore fixed wind infrastructure at the current construction levels is emerging and emphasizes that these processes must be considered at a regional scale for impact assessment.
Expansion of the OW sector to deeper waters is predicated on development of floating foundations, which are finite in depth and anchored to the seabed. Finite depth structures induce complex dynamics within stratified fluids, especially if structures intersect sharp density gradients (Dorrell et al., 2022). Although the sub-grid parameterization of the foundations can be tuned for floating foundations (by calibrating the Cd and c4 parameters), no published literature is available on the impact of floating foundations.
ii. Wind-wake effects
Earlier studies started to investigate the oceanic response to wind stress anomalies in idealized model approaches (Broström, 2008; Paskyabi & Fer, 2012; Paskyabi, 2015). The studies demonstrated the occurrence of an elevation pattern and corresponding up- and down-welling at the sea surface, which results from the changes in wind-driven Ekman transport. The resulting vertical transport, with vertical velocities of the order of m/day (with wind speed of 5 - 10 m/s), induces perturbations of the pycnocline and can influence the temperature and salinity distribution in a stratified water column (Broström, 2008; Ludewig, 2015). Less wind stress at the sea surface also causes decreasing horizontal surface currents behind OWFs, which are of the order of centimeters per second (Ludewig, 2015).
These studies formulated prerequisite conditions for the generation of OWFs wind-wake induced upwelling/downwelling dipole: (i) the characteristic width of the wind-wake has to be at least the internal radius of deformation (Broström, 2008); (ii) an almost constant wind direction for at least 8 - 10 h with moderate speeds 5 - 10 m/s (Ludewig, 2015).
Few empirical data of the wind-wake effects on the ocean dynamics are available, Floeter et al. (2022) provided observations of the existence of the upwelling/downwelling dipoles, using a TRIAXUS (a remotely operated towed vehicle, ROTV). In the summer stratified area of the North Sea, a distinct structural change in mixed layer depth and potential energy anomaly inside the wind-wake area of the OWFs was shown over a distance less than 5 km and a diagonal thermocline excursion of 10 - 14 m over a dipole dimension of 10 - 12 km.
The changes in the surface mixed layer depth can influence the pelagic ecosystem. A shallowing of the summer mixed layer depth brings the lower regions of the thermocline, and with it, high concentrations of nutrients and phytoplankton upward into more illuminated water depth levels, and it can be expected that the phytoplankton organisms immediately increase their production.
Christiansen et al. (2022) used an observational-based empirical approach to parameterise the atmospheric wakes in a hydrodynamic model. Due to the vertical changes of the wake pattern (Frandsen et al., 2006; Emeis, 2010; Fitch et al., 2012; Akhtar et al., 2021), the empirical and analytical models that have been developed to parameterise the down-stream wake effects in the atmosphere in the past cannot directly be used for processes near the sea surface boundary (Christiansen et al., 2022 and references therein). Christiansen et al. (2022) adjusted former model assumptions using Synthetic Aperture Radar measurements so that they become applicable for numerical ocean modeling. The wind reduction formulation is based on earlier studies from Frandsen (1992), (2006) and alters the undisturbed wind field by the wind speed deficit. The deficit consists of two components describing the downstream wake recovery, described by an exponential decay function, and the width of the wake structure, described by a symmetric exponential function scaled by the wind farm width. Christiansen et al. (2022) generated wakes with constant dimensions, which, in terms of the different wind farm properties (e.g., the number of turbines and turbine density) or the changing atmospheric conditions, lead to over- and under-estimation of the actual wake dimensions.
The wind reduction is on average around 1 - 2% relative to the mean undisturbed wind field and can reach even beyond 5% in areas of clustered wind farms. Both wind speed and wind stress exhibit continuous deficits over several tens of kilometres, which do not fully recover in between adjacent wind farms and are particularly strong in the case of superimposed wakes. The wake effects lead to unanticipated spatial variability in the mean horizontal currents and to the formation of large-scale dipoles in the sea surface elevation. Ultimately, the dipole-related processes affect the stratification development in the southern North Sea and indicate potential impact on marine ecosystem processes (further investigated in Daewel et al., 2022).
Spatial differences in the magnitude of wake effects occurred, despite similar changes in wind forcing. As further investigated in Christiansen et al. (2022b), tides and mixing play an important role in the changes in hydrodynamics causes by atmospheric wind farm wakes:
i. Tidal currents can deflect and even inverse wake-induced processes. The alignment between wind and tidal currents determines the magnitude of the wake effects, the periodic tidal currents can mitigate the impact of the wind speed reduction over time due to opposing changes in the horizontal flow, resulting in hydrodynamic changes only half as strong as those without tides. This tidal mitigation can translate into the development of sea level dipoles.
ii. Stratification conditions and tidal mixing rates determine the impact on vertical transport and density stratification. Changes are much more pronounced in stratified waters, whereas in well-mixed waters the impact of wake effects on the density distribution appeared weaker (tidal stirring attenuates the impact).
Different from earlier studies, Daewel et al. (2022) used a high-resolution atmospheric model (COSMO-CLM) including a parameterization of OWFs as a momentum sink and a source of TKE, which takes into account the size of the wind farm and the number of turbines (Akhtar et al., 2021) and estimates the impacts not only on the wind field but on the full atmospheric physics. The atmospheric model has been used to force a fully coupled physical-biogeochemical model, showing that the changes in atmospheric conditions will propagate through the ocean hydrodynamics and change stratification intensity and pattern, slow down circulation, systematically decrease bottom shear stress, increase sediment carbon, change primary productivity and dissolved oxygen distribution (Daewel et al., 2022).
Raghukumar et al. (2023) also used a high-resolution wind model, the Weather Research and Forecasting model with Wind Farm Parameterization (WRF–WFP) (Fitch et al., 2012), which represents WTs as a momentum sink and turbulence source, using turbine parameters such as hub height, rotor diameter, power curve, and thrust coefficients to calculate the magnitude of the source and sink terms (Lee & Lundquist, 2017). WRF is an established and validated operational weather model, WRF–WFP has been utilized in a number of studies to evaluate the effects of wind farms on mesoscale weather patterns (Jiménez et al., 2015; Huang & Hall, 2015; Eriksson et al., 2015; Raghukumar et al., 2022). The model accounts for turbine-turbine and wake-turbine interactions and thereby provides an accurate assessment of the wind field around an OWF (Churchfield et al., 2012). Raghukumar et al. (2023) found that wind speeds at 10 m height are reduced by approximately 5%, with wakes extending approximately 150 km downwind of the OWF. The wind fields (computed in the absence and presence of WTs) were used to force a regional ocean circulation model. It was found that the net upwelling, in a wide coastal band of the California coast, changes relatively little due the wind speed changes, but the spatial structure of upwelling within this coastal region can be shifted outside the bounds of natural variability. The consequences of these changes in physical upwelling structure on the ecosystem are currently unknown.
iii. Ongoing modelling efforts
PELAgIO and ECOWind-ACCELERATE (ECOWind programme) are developing/implementing numerical models for wave, hydrodynamics and biogeochemical predictions. These models will feed into a rapid evidence assessment of how climate change and OWF installation combine to impact seabed, water column, marine wildlife, and wider ecosystem structures. Local and regional scale models can simulate the interaction between offshore infrastructure and the environment in the near-field. UK shelf-wide models can provide insights on the cumulative effects of multiple offshore infrastructures (OWFs) and their interaction with the environment in the far-field. Particularly important is the integration of physical and biogeochemical modelling (hydrodynamics, sediment transport, water quality and productivity), as it is only in this holistic way that the full environmental impact can be understood. ECOWind projects are also combining climate projections of future storminess and sea-level rise: changing baseline against which OWF will also alter the environment.
Particle tracking simulations can yield information about connectivity between OWFs. OWFs provide a hard substrate habitat for benthic marine species which can spread between sites in their larval stage and can modify the natural ecological connectivity acting potentially as a source and sink of larvae: which means they can act as ‘stepping stones’ and have positive (enhancing resilience of protected species) or negative (spreading non-native invasive species) effects on the environment. Particle-tracking models simulations can help understanding how future planned OWFs or decommissioning of existing ones at the end of service, contribute to changes in larval connectivity.
A particle-tracking dataset and connectivity matrices has been developed in the CHASANS project (INSITE programme), the dataset can be interrogated to understand the connectivity of existing and future artificial structure in the northern North Sea for guidance on planning, decommissioning and derogation of man-made artificial structures.
As well as models dealing with specific physical processes, modelling approaches have also been used to represent bio-geochemical processes in OWFs (e.g. van der Molen et al., 2014) as well as trophic interactions (e.g. Halouani et al., 2020). For example, the Ecopath with Ecosim modelling package has been extensively used to model the response of both pelagic and benthic assemblages in OWFs. Halouani et al. (2020) use the Ecospace module within Ecopath to make spatially explicit predictions of spillover effects within, near, and far from OWFs. Trophic models, such as Ecopath, are parameterised using high-quality observation data, often provided by monitoring programmes. In the case of Halouani et al. (2020), observation data used in the parameterization of the model included fisheries data, satellite imagery, and reference values from the scientific literature. More recently, Pezy et al. (2020) also used nine Ecopath with Ecosim models to estimate the biological changes (inclusive of all trophic components from phytoplankton through to marine mammals) for OWFs spanning three sedimentary habitats at three temporal stages (winter, summer, and whole year). Following this exercise, Pezy et al. (2020) highlight the value of trophic modelling in OWFs for their ability to: (i) capture structural and functional change; (ii) merge and improve the interpretation of several sources of observational data; and (iii) capture, and meaningfully report on ecological complexity.
Bringing multiple models together offers an opportunity to capture more complexity. An example of a coupled model that integrated a physical-biogeochemical model with other physical models is well represented by the work of van der Molen et al. (2014). This study merged the output from a model wave, a physical-biogeochemical model (i.e. European Regional Seas Ecosystem Model), and an acoustic energy flux sound field model. The coupled model successfully estimated several physio-chemical and biological variables simultaneously for the study region containing a hypothetical OWF. One apparent issue with coupling so many models was the differing spatial resolution of the models and the representation of spatial scale for different processes. For example, noise propagation was the localised impact whereas the ecosystem impacts were estimated to be the most diffuse and spread over a larger area. This highlights a major difficulty in producing models for larger model domains as the working resolution needs to account for processes that occur at the finest spatial, and/or temporal, scale. This determines the working resolution of the model and may have significant implications for the computational power needed to run the model for large extents. Interestingly, the coupled model produced by van der Molen et al. (2014) did predict that the overall impact generated for a specific amount of energy collection would be less as turbines become progressively larger and more powerful. Predictions like this highlight the value of model outputs for the transferability of cause-effect mechanisms addressing future scenarios or new settings.
Other examples of coupled models include the merging of a hydrodynamic model with a microplankton-detritus model and dynamic energy model to estimate the local pelagic and benthic effects of blue mussels attached to OWF foundations and turbines (Maar et al., 2009). Burkhard et al. (2011) coupled a bio-geochemical model (ERSEM), with Ecopath and a hydrodynamic model (MIKE21) to estimate the overall change in ecological integrity of an OWF in the German North Sea.
The examples of coupled models provided above all directly utilise large numerical or mechanistic models that are computationally demanding. By comparison, Pınarbaşı et al. (2019) used Bayesian Belief Networks to model the spatial distribution of OWF feasibility both in the Basque Country and for the North East Atlantic/Western Mediterranean. Bayesian Belief Networks are extremely flexible models that can accept various inputs and formats (including expert judgement, spatial data, and large, quantitative datasets). Furthermore, BBNs also incorporate estimates of uncertainty and can be used to estimate, and trace, the total propagated error through these models, For the BBN models used for the Basque Country, Pınarbaşı et al. (2019) were able to integrate: (i) technical and economic variables; (ii) three biological components (i.e. seabirds, marine mammals and macrobenthos); and (iii) ten other variables reflecting interactions with other anthropogenic activities and socio-economic factors. Pınarbaşı et al. (2019) highlight the value of BBNs for their ability to integrate many different sources of information within a probabilistic framework that is computationally quick to compile. Although spatially explicit estimates of OWF feasibility were estimated by Pınarbaşı et al. (2019), BBNs can be designed to simulate any number of response variables including specific ecological impacts, marine net gain, functional indices, or total ecosystem service provision. Given their flexibility, it is clear when several of the ECOWind projects (i.e. PELAgIO and BOWIE) have adopted the use of BBNs for considering tropic cascades/combined effects and in-combination impacts (i.e. pressures from multiple activities on multiple receptors).
Finally, ecosystem services can then be classified to understand the costs and benefits of OWF installations. Models can ultimately be designed to interface with socio-economic systems. For example, Haraldsson et al. (2020) used a Social-Ecological-System model to estimate the impacts of the future expansion of OWFs in the Eastern English Channel. The ecological subsystem of the model included nine biological components. The social subsystem has six sectors (e.g. tourism, fisheries, OW industry). Ultimately, the Social-Ecological-System model estimated ‘perceived environmental quality’, ‘perceived well-being’, and the loss or gain experienced by each industrial sector. Haraldsson et al. (2020) concludes that these types of coupled models are particularly helpful for representing the complexity inherence in environmental systems that also include socio-economic considerations. Furthermore, the Social-Ecological-System was found to capture both indirect and unexpected changes within the modelled domain.
Contact
Email: ScotMER@gov.scot
There is a problem
Thanks for your feedback