Offshore wind - birds on migration in Scottish waters: strategic review
A report detailing the migratory routes of bird species around the UK and Ireland which have the potential to be impacted by offshore wind developments. This forms part of the strategic study of collision risk for birds on migration and further development of the stochastic collision risk modelling tool work package one.
Approach to baseline data update
Population estimates
The first step in assessing migrant collision risk is defining the size of the population passing through UK waters. We provided population estimates at three levels: 1) The biogeographic (flyway) population estimate, to provide context for the size of the population passing through UK waters; 2) the UK population size; and 3) the size of the population passing through UK waters, including those individuals either breeding and/or wintering in the UK, as well as individuals on passage from elsewhere in the flyway (either within the UK or elsewhere).
We extracted biogeographic (flyway) population estimates for each species used to inform the report on the Conservation Status of Waterbirds in the African-Eurasian Waterbird Agreement area (8th edition) (AEWA, 2021) from the Wetlands International Waterbird Population Estimates (Wetland International, 2021), and for non-waterbird species from Birdlife International (2015), or other sources where appropriate. The biogeographic estimate includes all subspecies and/or populations with meaningful numbers either breeding, wintering or on passage in the UK.
UK bird populations are the subject of intensive national monitoring schemes such as the Breeding Bird Survey (BBS) and Wetland Bird Survey (WeBS), as well as some species-specific schemes. Periodic assessments of the size of breeding and wintering bird populations in the UK and in Great Britain are made by the Avian Population Estimates Panel (APEP). We assessed the size of UK (Scotland, England, Wales and Northern Ireland) populations, extracting breeding and wintering estimates for each species in Table 1 from the most recent population assessment (APEP4; Woodward et al. 2020), where these were available.
For both biogeographic and UK population estimates, where estimates were given in a unit other than 'individuals' (i.e., pairs, males, females, nests), the total number of individuals was calculated by multiplying the estimate given by two. Furthermore, confidence in population estimates is given according to that in the latest population assessment (Wetlands International, 2021; BirdLife International, 2021; APEP4, 2020).
For each species, we then used information on migratory routes as well as expert knowledge to estimate the percentage of individuals (either breeding, non-breeding, or passage) from the relevant biogeographic population that are likely to move through UK waters. However, in many cases, these estimates are likely to be highly uncertain. Consequently, we assessed our confidence in percentage estimates based on the quality of published information and expert knowledge of the species' migration routes (see Table 3 for confidence estimate criteria).
Table 3. Confidence estimates for migration routes, timing of migration, flight heights and flight speeds were assessed according to the general subjective criteria given below.
Low
Data deficient, small sample sizes, low certainty that data are representative of the population, poor spatial resolution. Given there can be strong similarities in the flight behaviours of closely related species (e.g. Alerstam et al. 2007), this includes cases where parameter values based on data from closely related species have been recommended as no species-specific data were available.
Medium
Some data available, medium sample sizes, certainty that data are representative of part of the population, medium spatial resolution.
High
Good data available, large sample sizes, certainty that data are representative of the majority of the population, high spatial resolution.
Migratory routes
The migratory routes taken by species through UK waters is highly uncertain in most cases, apart from larger species where tracking data is increasingly available for some populations. Previous attempts to assess the risk posed to migrants by offshore wind farms (e.g., Wright et al. 2012) have used a broad-scale approach based on UK ringing data from the Migration Atlas (Wernham et al. 2002) and the limited tracking and radar data that were available at the time. The current project uses this same approach but incorporates additional information from new ringing data as well as new tracking and radar studies published since Wright et al. (2012).
Migration zone maps based on ringing data
Marine migration zones were mapped for all species. For a given species, maps were generated for marine migration zones of the SPA-relevant populations of that species. For example, if UK SPAs are designated for breeding populations of that species, then a map was generated of a) the migration zones of the UK breeding population of that species; and b) the migration zones of populations of that species breeding outside the UK in the wider biogeographical area (northern and western Europe and Iceland) and passing through UK waters. Some species have UK SPAs designated for them for multiple seasons (e.g., breeding and wintering); for these species, one map was generated for each season for which a species has an SPA designated. For a few species with both breeding and wintering SPA populations, there was very little information on migration routes and so breeding and wintering population migration zones were combined into a single map (e.g., Slavonian Grebe Podiceps auritus).
For two of the study species selected by the Project Steering Group, no UK SPAs are designated. The relevant season was defined for these species based on their frequency of occurrence and abundance in the UK: breeding for Montagu's Harrier (Circus pygargus), and winter and breeding for White-tailed Eagle (Haliaeeatus albicilla).
The spatial domain of the migration zone maps included UK waters, plus the facing coasts of northern and western Europe, and Iceland if appropriate.
Migration zone maps were generated by taking the migration zone maps from Wright et al. (2012), splitting the migration zones into UK and non-UK populations, and updating them to take more recently available data into account. Three species (Great Northern Diver Gavia immer, Black-throated Diver Gavia arctica and Spotted Crake Porzana porzana) were not included in Wright et al. (2012); migration zone maps were generated for these species following the methodology set out in Wright et al. (2012).
Migration zones through UK waters were determined by establishing the breeding or wintering grounds of the species using IUCN range data. Migration zones were further refined using: 1) ringing recovery location data for birds ringed in the UK & Ireland; 2) information on foreign-ringed birds from Wernham et al. (2002); and 3) GPS tracking data, where available.
Considerable uncertainty in migration routes remains for many species (e.g., the wintering location of Icelandic Shelduck is not known). Both ringing and tracking data can be spatially biased, if ringing and tagging effort is spatially uneven. Uncertainty in the migration zones is noted in the text accompanying the maps. Confidence in migration routes was assessed using the criteria given in Table 3. However, confidence in migration routes based only on ringing data is generally considered 'Low' despite large numbers of ringing recoveries being available for some species, as the ringing data only show connectivity between two or more locations and do not show the migration route followed. Confidence in tracking data is usually considered 'Medium' or 'High' depending on the robustness of the tracking method used and on whether data from small tracking samples are supported by ringing data.
Tracking data
The expansion of tracking technology means that, for some species, it may be possible to reduce uncertainty in migration routes. For each of the species listed in Table 1, we conducted a systematic primary literature review to identify any further evidence which could refine the broad migratory corridors given in Wright et al. (2012) and WWT (2014). We searched ISI Web of Knowledge and Google Scholar for studies between 1990-2021 using search terms selected to identify studies on the relevant species (scientific and common names, as well as collective terms i.e., duck, goose, wader etc), topics (Migrat*, Telemetry, Track*, Route, GPS, Tag*, Displace*, Colli*) and geographic area (UK). Suitable studies pertained to the correct species or species group, geographic area (Scotland through to Europe), season (includes migration information), and contained tracking information. A further search was made in Movebank[9] to identify any publicly available tracking datasets. For species where only summarised migration route information was available from published studies (e.g., maps of tracks), these are discussed in the text.
In addition to this, project partners own or have access to tracking datasets relating to several of the species concerned (e.g., Shelduck, Pink-footed Goose, Osprey). For species with raw tracking datasets, 90% utilisation distributions were calculated for fixes over water, and presented on supplementary migration zone maps (see Appendix 1). For these species, additional maps were generated showing the full tracks.
In many cases, the sample sizes associated with tracking data are relatively small, and the implications of this are discussed in relation to reducing uncertainty in relation to assumed migratory corridors. Note also that tracking data is not necessarily representative of all age classes, and age class of tracked birds was either not considered or was unspecified by the data holder.
While tracking data can provide high spatial resolution information on individual movements, it is not necessarily representative of the entire UK population as a whole, with potential spatial and temporal biases in the deployment of tracking devices leading to misinterpretation of migration corridors. In species accounts, we therefore present the overall migration zones updated from Wright et al. (2012) based on ringing data, while including a description of the information provided by tracking data in the text. For species with available raw tracking data, kernel density distributions based on tracking data are superimposed on the migration zones, with these maps provided in Appendix 1.
Timing of migration
The timing of migratory movements determines the migration risk period for the population passing through UK waters and has been estimated using data collected through BirdTrack[10]. We first obtained GIS geometries of the relevant marine planning regions for each country. Using shapefiles for the 11 Scottish Marine Regions[11] and 6 Marine Plan Areas in England[12], we created a coastal buffer region in Scotland and England extending 20 km inland. For Wales and Northern Ireland, we created country-level 20 km coastal buffers from an all-UK polygon (see Figure 3). For Scotland and England, two further shapefiles were created by merging the separate marine plan areas together into single, country-specific geometries.
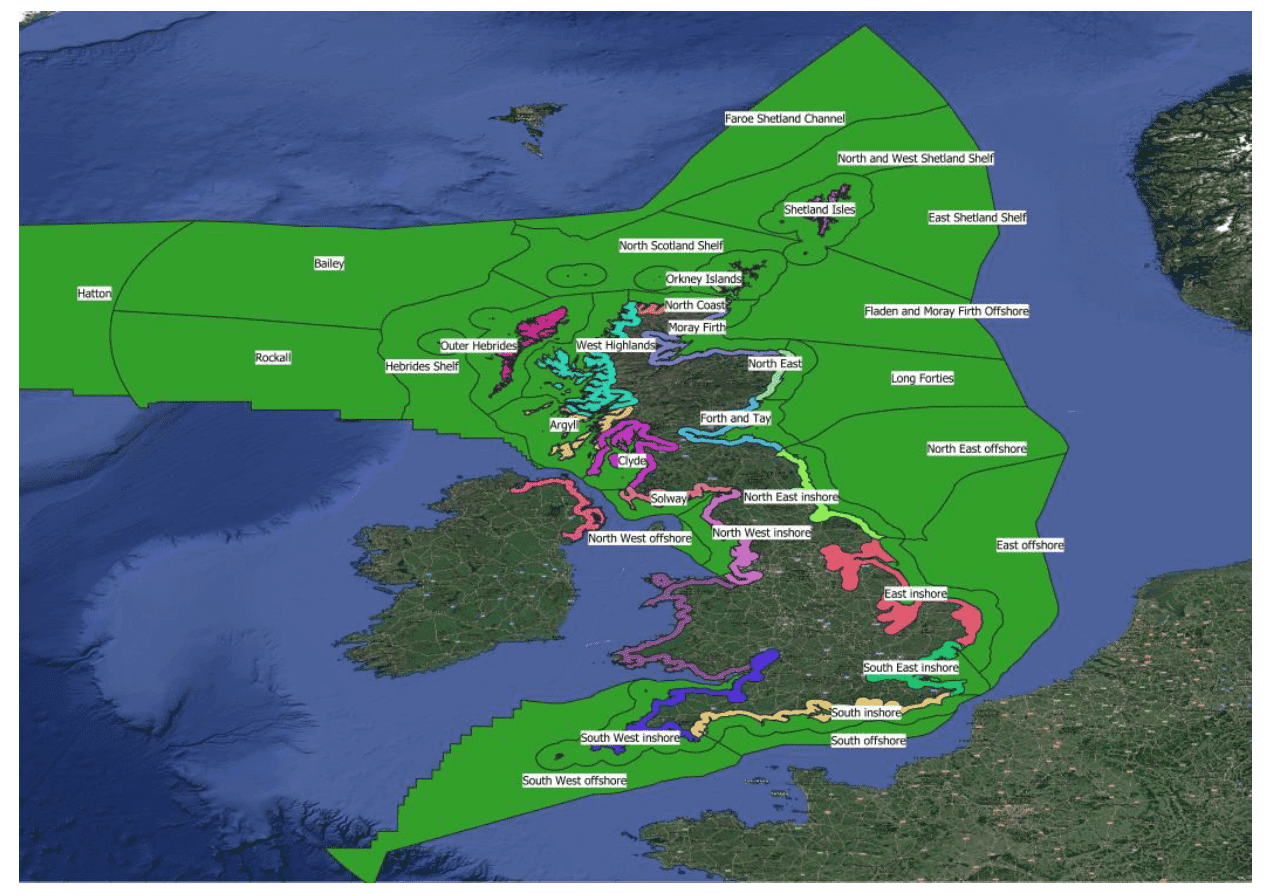
BirdTrack data for marine plan regions as well as all-country regions were extracted between 2010–2019 for each of the focal species, with the mean ± 95% confidence limits reporting rate from complete lists in these regions across the time period calculated for each week throughout the year.
The data for each species were then plotted per region (see Appendix 2), showing the mean ± 95% confidence limits and a locally estimated scatterplot smoothing ('loess') regression line (example for Pink-footed Goose Anser brachyrhynchus, Figure 4). The peak migration timings for each region were extracted based on visually determining the weekly location of turning points on the loess curve bracketing rates of change in reporting rate, signifying the start and end of movement periods.
The identified migration periods were then plotted onto these graphs to highlight the peak migration risk periods. The graphs are arranged in geographical orientation to aid interpretation of how the timings of migration onset/peak/end vary across the country.
Confidence in migration timings was assessed based on the criteria given in Table 3, with confidence levels higher in cases where more robust data were available (e.g. tracking data). BirdTrack data were generally classified as 'Low' or 'Medium' confidence depending on whether or not the patterns shown in the graphs were clear and consistent and hence clearly likely to be representative of at least part of the population. In general, there is lower confidence in the timing of movements for species with different individuals visiting the UK as winter or breeding migrants, including some duck species and breeding waders.
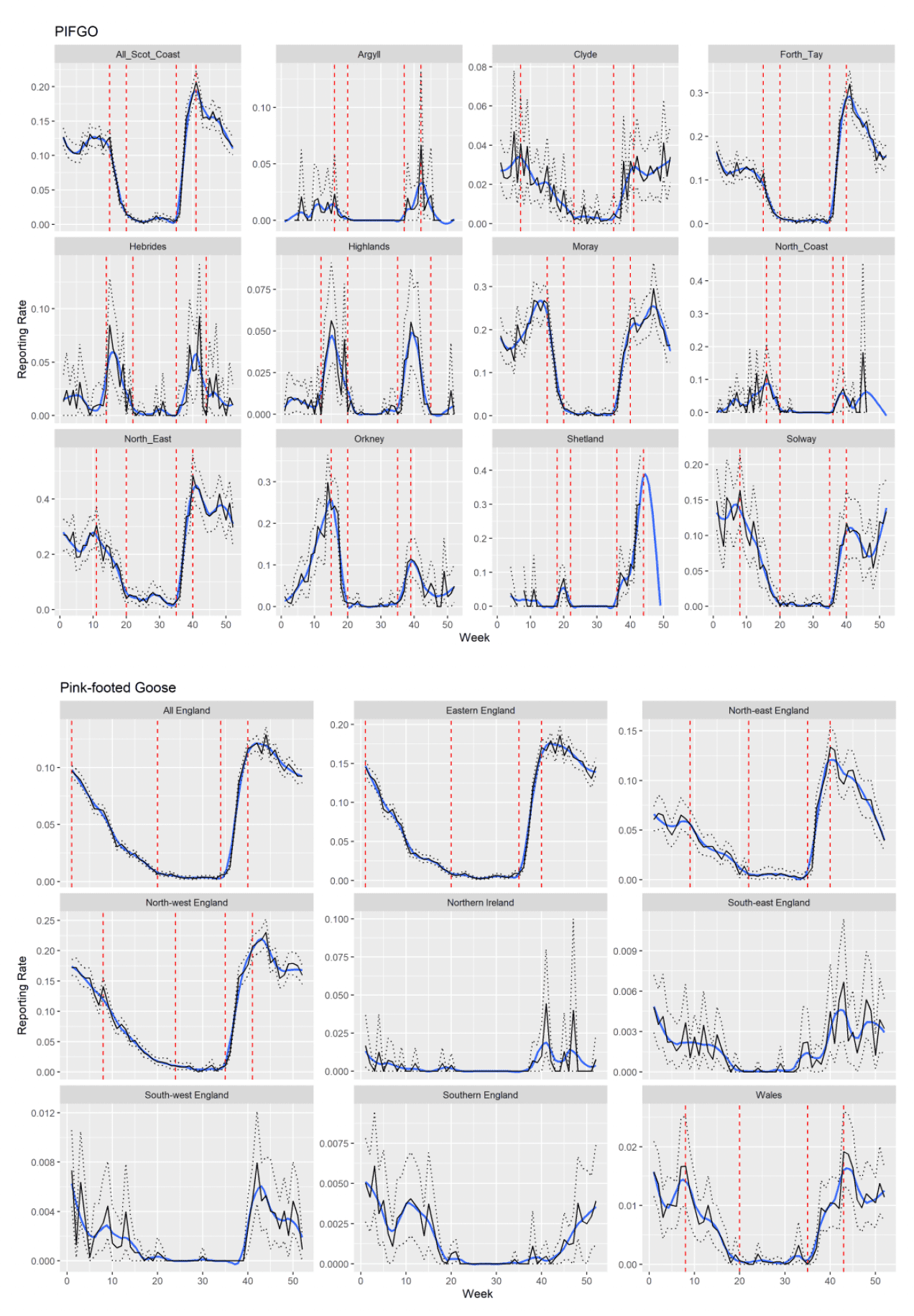
For most species, only the main migratory periods will be highlighted, although in some cases, moult migration movements will be identifiable from the BirdTrack reporting rate graphs and will be discussed in the text. Cold weather movements will be much more difficult to identify as the variability in timing of these between years means that a 10-year average is unlikely to capture obvious patterns. We also consider and discuss any spatial patterns in the timing of migration by comparing reporting rates between regions.
Migration flight heights
The height at which birds migrate over the sea plays a crucial role in determining their exposure to collision risk. Previous reviews (Wright et al. 2012, WWT 2014) highlighted significant uncertainty surrounding assessments of the flight heights of migrating birds and, consequently, assigned them to broad flight height categories. Since then, studies making use of technology such as radar (e.g. Fijn et al. 2015) and GPS tags (e.g. Griffin et al. 2016) have been used to measure the heights of migrating birds. We conduct a brief review of existing evidence on flight heights, alongside an assessment of our confidence in these estimates (using the criteria given in Table 3).
For each species, we searched Google Scholar and Web of Science with the terms 'flight height' with the species' scientific name. We also tried 'flight altitude', but it returned the same results as 'flight height'. For Google Scholar, we used relevant references from the first two pages of search results, for each species, together with additional references containing flight height estimates mentioned in these references. We then extracted all flight height estimates from this set of references. We ignored display flights, and omitted spring estimates from Green & Alerstam (2000), as they appear to be from a subset of the dataset in Green (2004). Some flight height estimates from (Krijgsveld et al. (2011) and (Skov et al. 2012) were extracted from figures using the WebPlotDigitizer tool[13].
Fitted flight height distributions were available from Johnston et al. (2014) for four relevant species. Mean flight height was calculated from these distributions by taking the sum of modelled proportion of flights in each height band * midpoint of the height band.
Reflecting uncertainty surrounding turbine sizes in future consenting rounds, we consider collision risk height to range from 22m a.s.l. (the minimum rotor clearance permitted) to approximately 300m a.s.l., reflecting a precautionary estimate for the maximum rotor height of an 18MW turbine[14]. Given the data presented in studies of the flight heights of migrating birds, it is often not practical to define an exact proportion of birds at collision risk height, particularly when the exact dimensions of turbines may be unknown. With this in mind, based on the data presented we assign the proportion of birds at collision risk height to rough categories of 0, 25, 50, 75 or 100% with reference to the published estimates of species mean, median and range of flight heights. Where no data are available, we assume that 100% of birds are likely to be flying at collision risk height, and confidence in the presented values is assessed as low. In other situations, the % of birds estimated at collision risk height is given with low, medium, or high confidence with reference to the categories in Table 3.
Migration flight speeds
The existing sCRM is highly sensitive to bird flight speed as it is used twice within the model, firstly to estimate the flux rate and, secondly to estimate the probability of a bird colliding with a turbine (Masden et al. 2021). However, for the migration collision risk model, the number of birds passing through the turbine rotor swept areas (flux rate) is estimated with reference to the total size of the population concerned, and each bird is assumed to pass through each wind farm only once during each migration. Consequently, in assessing migrant collision risk, bird flight speed is only used within the model, to estimate the probability of a bird colliding with a turbine. This means that any migrant collision risk model is likely to be less sensitive to assumptions about bird flight speed.
We conducted a brief review of flight speed for the species listed in Table 1. For each species, we searched Google Scholar and Web of Science with the terms 'flight speed' and the species' scientific name, and then again with the species' common name, and used relevant references from the first two pages of search results, for each species, together with additional references containing flight speed estimates mentioned in these references. We then extracted all flight speed estimates from this set of references.
We omitted the following studies: any from wind tunnels as these did not involve free-flying birds; metrics of flight speed which did not involve direct measurement (e.g. comparing a flock of birds to the known speed of a passing train), except for Merlin, for which we could find no other flight speed estimates; specific studies which were returned by the search results but which were all included in Alerstam et al. (2007); Gudmundsson et al. (1995) as it gave estimates for birds flying over the Greenland icecap rather than at sea; any 'pers. comm.' flight speed estimates.
Collision Risk Models typically make use of groundspeed rather than airspeed (Masden et al. 2021). Consequently, we extracted groundspeed where available, but airspeed otherwise, noting that except in extreme cases, the two values are likely to be similar. Because flight speed often differed significantly between seasons, either due to prevailing wind, or the need to arrive earlier in the spring to obtain optimal breeding habitat (e.g., Nilsson et al. 2013), we accounted for season wherever possible while extracting data. We give values as close to the global mean as possible, as sometimes means were given within subgroups rather than as a global mean (e.g., flight mode, season, individual), though with preference for flights over sea, where available.
Flight speed was estimated from raw tracking data where available, by calculating distance/time between fixes. Per bird or track, distance was divided by time between consecutive fixes if: both of those fixes were over sea; distance and time were both > 0; distance was > 5 km (to avoid spurious movements from position error) and < 200 km (the further apart the fixes are, the more a straight line will deviate from a realistic bird track).
Given the range of approaches to measuring flight speeds, different sample sizes between these and differences in the availability of estimates from migration and non-migration seasons, it was not possible to adopt a consistent approach to providing recommended values for collision risk modelling. Where possible, we have prioritised direct measurements (e.g., using radar or GPS) during migratory periods and studies with the largest sample sizes. We could not find any flight speed estimates for Corncrake, Great Crested Grebe, Stone-Curlew, Avocet, Golden Plover, Dotterel or Purple Sandpiper. Flight speeds for a closely related species have been recommended for these seven species and the overall confidence score has been set to 'Low'.
Avoidance rates and behaviour
Collision risk models are known to be particularly sensitive to estimated avoidance rates (Chamberlain et al. 2006; Cook et al. 2018; Masden et al. 2021). Whilst avoidance behaviour can be thought of as a series of decisions made by a bird along a continuum as it approaches a turbine, in practice it is usually broken down into three distinct spatial scales – macro, meso and micro – reflecting both the behaviour driving any avoidance response and, the limitations imposed by the methodologies available to quantify it (Cook, et al. 2018; Cook et al. 2014; May, 2015). Whilst approaches such as the use of radar are enabling us to better quantify macro-avoidance (avoidance of the wind farm as a whole) (Desholm & Kahlert, 2005; Plonczkier & Simms, 2012), quantifying meso-avoidance (avoidance of turbines within a wind farm) and micro-avoidance ('last-second' action to avoid a collision) remains challenging. Reflecting this, a fourth category of "within-windfarm" avoidance is used based on a comparison of the number of collisions recorded and the number of collisions expected in the absence of any avoidance action (Cook et al. 2014; Cook et al. 2018).
Two approaches were used to derive estimates of avoidance rates for migrating birds. Firstly, we reviewed existing guidance documents and other evidence. Secondly, we estimated avoidance rates through the comparison of observed and predicted collision rates.
Review of existing guidance and evidence
Given the high profile of collisions between birds and wind farms in the consenting process for both onshore and offshore developments, there is a substantial body of existing literature from which to draw. We used these guidance documents (Cook et al. 2014; SNH, 2017) as the starting point for our review, going back to the sources cited in support of this guidance in order to extract the relevant values and assess the quality of the evidence. We then broadened our search in order to identify published studies which described interactions between the key species and onshore or offshore wind farms. This included those where data had been collected by visual observers (e.g Krijgsveld et al. 2011), radar (e.g Desholm & Kahlert, 20 05), boat/aerial survey (Mendel et al. 2019) and GPS (Schaub et al. 2020). Several studies reported the proportion of tracked birds entering windfarms (Desholm & Kahlert, 2005; Plonczkier & Simms, 2012). Whilst this is useful information as it gives an indication of the likely numbers passing through developments, these cannot be thought of as avoidance rates as they do not account for birds whose flightpaths would not have intersected with a wind turbine.
Table 4. Confidence estimates for avoidance rates were assessed according to the criteria given below.
Very Low
Comparison of observed and predicted collision rates at sites with low levels of bird activity; estimates based on expert opinion (e.g., lack of observed corpses).
Low
Data collected using direct measurements but not treated in a statistically robust manner (e.g., do not account for flightpaths that would not intersect with windfarm); comparison of observed and predicted collision rates at sites with significant levels of bird activity.
Medium
Data collected using direct measurements (e.g., radar or GPS) and analysed in a statistically robust manner.
From each study, we extracted data describing the response of birds to a windfarm and considered whether the reported values reflected macro-, meso-, micro-, within-windfarm- or total-avoidance. In contrast to the previous parameters (Table 3), we did not feel that any of the datasets were sufficiently robust for confidence in the evidence presented to be assessed as "high". Whilst for some species, there was consistent evidence of macro-avoidance behaviour from radar (e.g. Desholm & Kahlert 2005; Plonczkier & Simms 2012), or post-construction monitoring data (e.g. Mendel et al. 2018), converting these values into avoidance rates suitable for use in collision risk models is challenging. Consequently, in these instances, evidence for avoidance behaviour was assessed as medium confidence. In other cases, the available evidence was derived from collision monitoring studies at onshore wind farms. Uncertainties in these data mean that the evidence available for avoidance rates in many species is far less robust than the evidence available to inform flight speeds or heights. Consequently, in these cases, we assessed confidence in the available data as being "very low". Descriptions of the categories used to describe confidence in the data available to quantify avoidance rates and behaviour are presented in Table 4.
Comparison of predicted and observed collision rates
Avoidance rates can be predicted by comparing observed collision rates with those predicted in the absence of any avoidance action. Estimating avoidance in this way requires an estimate of the total number of collisions recorded within a wind farm over a given time period and, an estimate of the rate at which birds move through the wind farm over that time period (Band et al. 2007; Cook et al. 2014; Scottish Natural Heritage, 2010). Based on the information presented in the above review and, a search for post-construction collision monitoring reports, we identified 31 sites covering onshore wind farms in the UK, USA, Europe, and Japan for which data describing both collision rates and passage rates involving species relevant to this review were reported. The studies identified by this review are listed in Appendix 3.
Avoidance rates for each species and group were then estimated following the approach set out in Cook et al. (2014) and described below:
1. As a first step, the hourly number of birds passing through the wind farm was estimated by dividing the total number of birds recorded during surveys by the total duration of these surveys.
2. The total number of birds passing through the wind farm over the duration of each survey was then estimated by multiplying the hourly passage rate by the total number of hours covered by each survey period (Eq. 1). Unless stated, nocturnal activity was assumed to be 0 for each species. When estimating avoidance rates, this is a precautionary assumption as it reduces the number of birds estimated to be at risk of collision, and therefore the predicted collision rate which is compared to the observed collision rate when estimating the overall avoidance rate (see Eq. 3). The total number of hours daylight and night over each survey period was estimated following the approach of Forsythe et al. (1995).
Equation 1
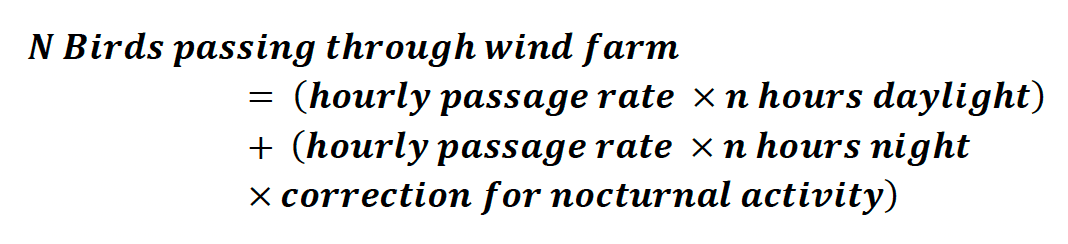
3. The vertical area of the survey window was estimated by multiplying the width of the survey window by the height of the survey window. Where no estimate of the height of the survey window was given, the maximum tip height of the turbines was used.
4. This was then multiplied by the total turbine frontal area as a proportion of the total survey frontal area (Eq. 2) to give an estimate of the total number of birds passing through the turbine rotor swept area.
Equation 2
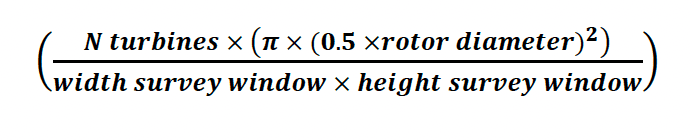
5. This was corrected by an estimate of the proportion of birds reported at collision risk height. Where the proportion of birds at risk height was not reported by a survey, this was based on the rotor diameter as a proportion of the height of the survey window, assuming birds were evenly distributed.
6. To get the number of collisions expected in the absence of avoidance, this figure was multiplied by the probability of collision estimated following Band (2012).
7. Finally, this was compared to the number of collisions recorded at each site (corrected for search area, scavenger activity and searcher efficiency where possible) using Eq. 3.
Equation 3

For each species and species group the process set out in Cook et al. (2014) was followed to estimate an avoidance rate across all years and sites using ratio estimators (Cochran, 1977) and the Delta method (Powell, 2007) to estimate the standard deviation and 95% confidence intervals around this figure.
At many sites, the focus of monitoring was on larger birds and protected species, meaning that passage rates for smaller birds, especially passerines were often not recorded. Similarly, as surveys were restricted to daylight hours, nocturnal species such as owls and nightjars, and any nocturnally migrating species during migration periods are likely to be systematically under-recorded. Consequently, values reported for nocturnal migrating species, as highlighted in the species accounts below, should be treated with caution. Five of the species considered in this review migrate only or mostly at night (Nightjar Caprimulgus europaeus, Corncrake, Spotted Crake, Great Crested Grebe Podiceps cristatus and Slavonian Grebe).
Results
Drawing from data presented in the studies listed in Appendix 3, and based on the above analyses, it was possible to estimate avoidance rates for 19 species, or species groups (Table 5). Given the uncertainty in much of the data underpinning this analysis, confidence in these values is assessed as very low. Where estimates are based on a limited number of sites and/or low passage rates, these are considered unreliable and have not been presented. Where possible, recommended avoidance rates reflect the appropriate species or group specific rates presented in Table 5. For species where group specific rates were not available (Spotted Crake, Corncrake, Nightjar, grebes, and divers), consideration was given to evidence for macro- and or meso-avoidance from other studies, and the ecology of the species concerned when selecting between a generic all-birds avoidance rates, or one from another species group. In the case of divers, although specific avoidance rates were not available, there was good evidence for strong macro avoidance which informed the recommended avoidance rate and the assessment of medium confidence in the values for this group.
Species/Group |
Avoidance Rate |
SD |
|
---|---|---|---|
Species-specific |
Mallard |
0.9801 |
0.00319 |
Golden Plover |
0.9999 |
0.00004 |
|
Greylag goose |
0.9996 |
0.00005 |
|
Pink-footed goose |
0.9999 |
0.00002 |
|
Whooper swan |
0.9874 |
0.00138 |
|
White-tailed eagle |
0.9872 |
0.00192 |
|
Group |
Falcon |
0.9891 |
0.00033 |
Hawk |
0.9956 |
0.00014 |
|
Eagle |
0.9972 |
0.00023 |
|
Ducks |
0.9851 |
0.00088 |
|
Heron |
0.9928 |
0.00092 |
|
Wader |
0.9996 |
0.00002 |
|
Goose |
0.9998 |
0.00001 |
|
Gamebird |
0.9875 |
0.00174 |
|
Swan |
0.9885 |
0.00091 |
|
All raptors |
0.9957 |
0.00006 |
|
Geese & swans |
0.9998 |
0.00001 |
|
Ducks, geese & swans |
0.9995 |
0.00001 |
|
All birds |
0.9954 |
0.00002 |
Contact
Email: ScotMER@gov.scot
There is a problem
Thanks for your feedback